Donald Trump wrestling with police officers during his arrest, Pope Francis walking around in a luxury puffer jacket, two images you've probably seen, but which don't represent scenes that actually took place in reality.
Manipulation of photographic media is not new – in fact, as Eric Benoist explains in his latest report the first “face swap” montage can be traced back to the late 1860s, when, post-assassination, the face of President Lincoln was affixed to John Calhoun’s body in order to produce a more “heroic” representation. While new it may not be, advances in generative technologies mean that it is more convincing than ever; indeed, the human eye is no longer able to discern artificially created imagery.
Similarly, the music industry has been grappling with a rise of deepfakes in the audio world – some of the more impressive and convincing tracks include Johnny Cash’s rendition of Barbie Girl and Frank Sinatra’s version of Gangsta’s Paradise.
The video world has likewise been faced with an invasion of artificially generated imagery. To discover just how convincing these can be, the now infamous 2018 Buzzfeed video created by Jordan Peele imitating President Obama makes for a compelling watch. Or, for a more amusing demonstration of the technology, Miles Fisher’s TikTok channel dedicated to deepfake videos of Tom Cruise is certainly entertaining.
While these examples err on the more lighthearted side, the technology is being used for nefarious purposes too.
We spoke to Eric Benoist to find out more about generative AI deepfakes and what the future holds.
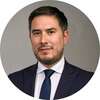
Eric Benoist, Tech and Data Research Expert, Natixis Corporate & Investment Banking
Perhaps a good place to start would be to understand exactly what a deepfake is?
Simply put, a deepfake refers to an image, video or recording that has been convincingly altered, manipulated, or entirely generated from scratch to misrepresent someone as doing or saying something that wasn’t actually done or said.
The technology, which first appeared in 2017, uses AI and sophisticated neural networks to generate ultra-realistic content. With enough data, it can create almost perfect imitations, making it very difficult to distinguish between real and fake.
There are various forms of deepfake spanning both visual and audio formats, and each variant is associated with a specific machine learning model best suited to its characteristics.
We looked at some seemingly lighthearted examples of deepfakes in the introduction – why should we be more wary about the way the technology is being used?
At today’s juncture, we can only assume that the human eye – or ear – will soon no longer be able to distinguish between real and fake. This is already the case for static visual content, such as photographic portraits – a development that is both impressive and frightening.
As for audio and video, they are rapidly approaching the same level of realism.
Today, we can only assume that the human eye – or ear – will soon no longer be able to distinguish between real and fake.
On the political front and in times of military conflict, the technology is used to influence public opinion and sow confusion and chaos. Within days of the Russian invasion of Ukraine, a video of President Zelensky began circulating on social media asking Ukrainian soldiers to lay down their arms and surrender. In January, a few days before the New Hampshire primary, Democratic voters in the US received a fake phone call mimicking Joe Biden's voice, asking them to stay home and save their vote for the November elections, in a fraudulent attempt to derail the incumbent president’s re-election bid.
Equally destructive, deepfakes are used to create sexually explicit content featuring women who have not consented to their image or likeness being exploited - pop superstar Taylor Swift recently bore the brunt of this deplorable practice.
As the saying traditionally goes, “seeing is believing”, and in the absence of concrete, irrefutable proof that deep learning techniques have been deployed for manipulative purposes, doubt will always remain ingrained in the minds of the target audience. After all, the deepfake “excuse” could easily be used to cover up otherwise reprehensible behaviours or uncomfortable situations. More toxic side-effects may then occur when parts of the population progressively refuse to accept the facts as they are conveyed, claiming that deepfakes are behind stories that do not satisfy their beliefs or interests.
When placed in the wrong hands, deepfakes can clearly be used as weapons of mass destabilisation.
So, as deepfakes become increasingly difficult to recognise, how are we going to be able to trust what we see and hear?
With their rapid proliferation, detecting deepfakes is somewhat of an uphill battle. What is becoming ever clearer is that AI itself will eventually constitute the very last line of defense against the threats of an indistinguishable digital reality.
In the current state of our technological mastery, it is still relatively easy to spot a rigged video sequence, but this probably won’t be the case for very long.
Today, the detection of deepfakes relies mostly on the identification of spatial and temporal inconsistencies. Spatial errors, for example, arise from a lack of relevant training data, or from a poor understanding - at model level - of the laws of physics (think irregular or distorted body geometries, bizarre positioning and alignment of hands and fingers, abnormal head poses, incorrect lighting and distorted backgrounds).
Today, the detection of deepfakes relies mostly on the identification of spatial and temporal inconsistencies.... Generation techniques however continue to gain ground at an impressive pace and detection methods remain insufficiently accurate and reliable.
Likewise, just as digital cameras produce their own signature due to differences between sensors and electronic components, the models used to generate deepfakes often leave a "trace" owing to the specifics of their architecture – in this regard, forensic methods concentrate directly on detecting these artifacts or "fingerprints", some of which are clearly visible to the naked eye, while others require more subtle statistical evaluation in the frequency spectrum.
Generation techniques however continue to gain ground at an impressive pace and detection methods remain insufficiently accurate and reliable. Further research is evidently needed into more efficient models, capable of better generalising to new materials and withstanding advanced adversarial attacks.
Could blockchain play a role in certifying ‘real’ audio and visual content?
Potentially. Blockchain could certainly be exploited to verify the provenance of media content, but this won’t guarantee its legitimacy. Whoever submits the content first must do so in good faith and without malicious intent. While decentralised ledgers constitute excellent candidates for a more trustworthy dissemination of data and information, they cannot prevent the creation of harmful deepfakes.
Should we then expect a near future populated by deepfakes with no way of discerning reality - or is that an overly dramatic prospect?
In a year when half the world's population goes to the polls, the sharp growth in deepfake numbers, and the profound impact they may have, are clearly of concern.
Regulators are increasingly – if slowly – waking up to the threats, but much will have developed within the industry by the time an effective and balanced legal arsenal is in place.
More money is needed to build reliable systematic detection tools, but startups developing the solutions to defend against the most harmful excesses of the technology are left with very limited financial support when competing for the attention of investors.
More money is needed to build reliable systematic detection tools, but startups developing the solutions to defend against the most harmful excesses of the technology are left with very limited financial support when competing for the attention of investors more attracted by the commercial prowess of Large Language Models (LLMs).
Educating people on the topic is certainly an important part of the fight. But the fight goes on and is far from won, particularly as each new advance in deep-learning science brings seemingly limitless possibilities – both good and bad.